Max Bennett’s book A Brief History of Intelligence overlays AI development with evolutionary psychology and offers compelling evidence for the power of modular, layered cognitive systems. Cognitive hive AI (CHAI) takes these insights from nature and applies them to artificial intelligence, creating a flexible, modular paradigm for AI implementation.
Bootstrapping: Nature’s modular ascent to intelligence
Bennett unveils a fascinating process of cognitive bootstrapping over hundreds of millions of years. Increasingly complex forms of intelligence emerged through a series of modular improvements, each building upon the capabilities of the previous stage.
At the most fundamental level, more “primitive” organisms such as nematodes exhibit approach/avoid behaviors based on simple valence systems. These allow the organism to move towards beneficial stimuli and away from harmful ones, creating a direct mapping of sensory input to motor output. This foundational level of intelligence, while basic, forms the bedrock upon which more complex cognitive abilities are built.
As we move up the evolutionary ladder, we encounter the emergence of associative learning. This marked a significant leap forward, enabling organisms to form connections between different stimuli or between actions and their consequences. This capability introduced a basic form of memory and the ability to modify behavior based on past experiences.
The development of emotional processing in early vertebrates represents another crucial step in the bootstrapping of intelligence. With the emergence of the limbic system, organisms gained the ability to guide behavior through more complex affective states. This innovation allowed for more nuanced responses to environmental challenges and laid the foundation for motivation and goal-directed behavior.
The evolution of executive functions in mammals, facilitated by the development of the prefrontal cortex, brought about a quantum leap in cognitive capabilities. This advancement introduced the ability to plan for future events, engage in complex problem-solving, and exercise impulse control. These higher-order cognitive functions allowed mammals to navigate increasingly complex environments and social structures.
At the pinnacle of this evolutionary progression, we find the development of theory of mind, particularly in primates and humans. This sophisticated cognitive ability allows individuals to model and predict the mental states of self and others, enabling complex social interactions and cooperation. It forms the basis for our most advanced social behaviors and cultural developments.
The modular nature of CHAI allows organizations to mirror this evolutionary path of intelligence in their AI implementations. By adopting this approach, businesses can:
- Start with basic AI functions and gradually incorporate more advanced modules as needs evolve
- Scale AI complexity to match organizational growth
- Adapt flexibly to changing business requirements or technological advancements
- Achieve interoperability between modules, potentially leading to emergent capabilities
For example, a manufacturing company might begin with simple sensor-based quality control (akin to basic valence), then add modules for predictive maintenance (associative learning), and eventually incorporate advanced planning systems for supply chain optimization (executive functions). As the company’s needs grow more complex, they could even implement modules for modeling supplier behavior and market dynamics (theory of mind).
This modular, evolutionary approach to AI implementation offers several key advantages:
- Targeted deployment of specific cognitive capabilities
- Gradual scaling of AI complexity
- Flexibility to adapt to new challenges or opportunities
- Potential for emergent behaviors through module interactions
By embracing modularity, CHAI doesn’t just imitate nature’s design—it provides a pathway for AI to potentially surpass biological limitations, opening up new frontiers in artificial intelligence. As our understanding of both biological and artificial intelligence continues to grow, CHAI stands ready to incorporate new insights, pushing the boundaries of what’s possible in machine cognition.
The actor-critic model: Nature’s argument for specialized modules
Another insight from Bennett’s book is the emergence of the actor-critic model in advanced cognitive systems. This model separates the action selection process (the actor) from the evaluation process (the critic), allowing for more efficient learning and adaptation.
In biological systems, this separation enables:
- More nuanced decision-making
- Faster learning from experience
- Better handling of delayed rewards or complex environmental feedback
CHAI takes this concept further by allowing for multiple specialized modules that can act as various “actors” and “critics” within the system. For example:
- Multiple actor modules could handle different types of tasks (e.g., language processing, visual analysis, decision-making)
- Various critic modules could evaluate performance based on different criteria (e.g., short-term gains, long-term strategic goals, ethical considerations)
- A coordination module could manage the interplay between actors and critics, adjusting the system’s overall behavior based on feedback and goals
This multi-modular approach enables CHAI systems to:
- Handle complex, multi-faceted problems more effectively
- Adapt to changing circumstances by reconfiguring the relationships between modules
- Provide more transparent decision-making processes, as the contributions of different modules can be isolated and examined
The case for modularity in AI
Here’s our case for modularity in cognitive systems:
- Adaptability: Modular systems can evolve more quickly, adding or modifying specific capabilities without overhauling the entire system. In CHAI, this translates to easier updates and adaptations to new challenges.
- Efficiency: Specialization of modules allows for more efficient processing of specific tasks. CHAI can leverage this by activating only the necessary modules for a given task, potentially reducing computational overhead.
- Robustness: In biological systems, damage to one module doesn’t necessarily compromise the entire system. Similarly, CHAI’s modularity can enhance system resilience, as issues in one module can be isolated and addressed without disrupting the entire AI.
- Scalability: Nature scaled intelligence by adding new cognitive modules over time. CHAI mimics this by allowing organizations to start with essential modules and scale up as needed, aligning AI capabilities with business growth.
- Transparency: The modular structure of evolved intelligence allows scientists to study specific cognitive functions. In CHAI, this modularity can enhance explainability, as the contributions of individual modules to decision-making can be more easily traced and understood.
CHAI as the next step in AI evolution
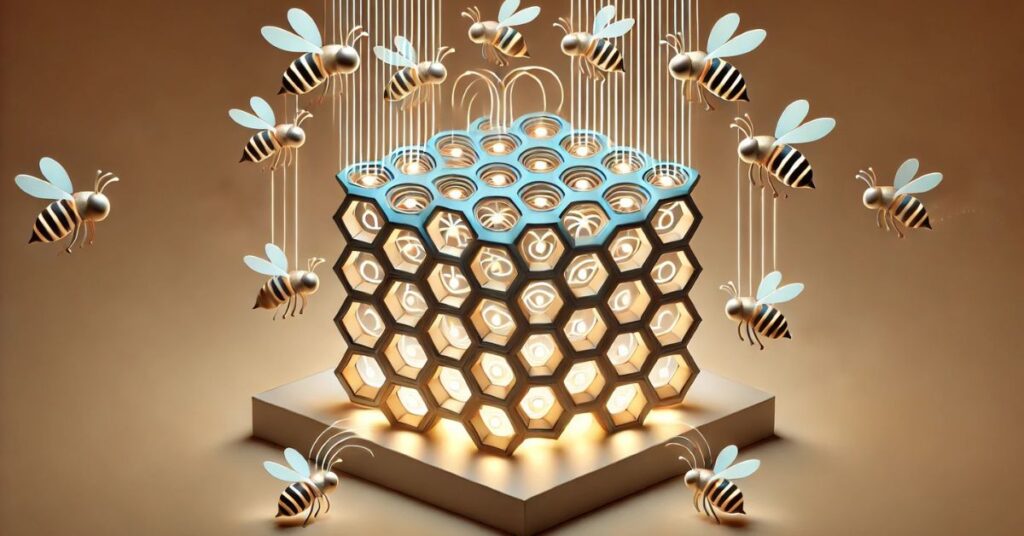
By adopting a modular paradigm inspired by the evolution of biological intelligence, CHAI offers a flexible, scalable approach to AI implementation. This approach allows organizations to:
- Tailor AI capabilities to specific needs
- Start small and scale up over time
- Adapt more quickly to changing requirements or new technologies
- Potentially enhance system robustness and transparency
As we continue to unravel the complexities of natural intelligence, CHAI stands ready to incorporate new insights, pushing the boundaries of what’s possible in artificial intelligence. By embracing modularity, CHAI doesn’t just imitate nature’s design—it provides a framework for AI to evolve beyond the limitations of biological systems, opening up new frontiers in machine cognition.